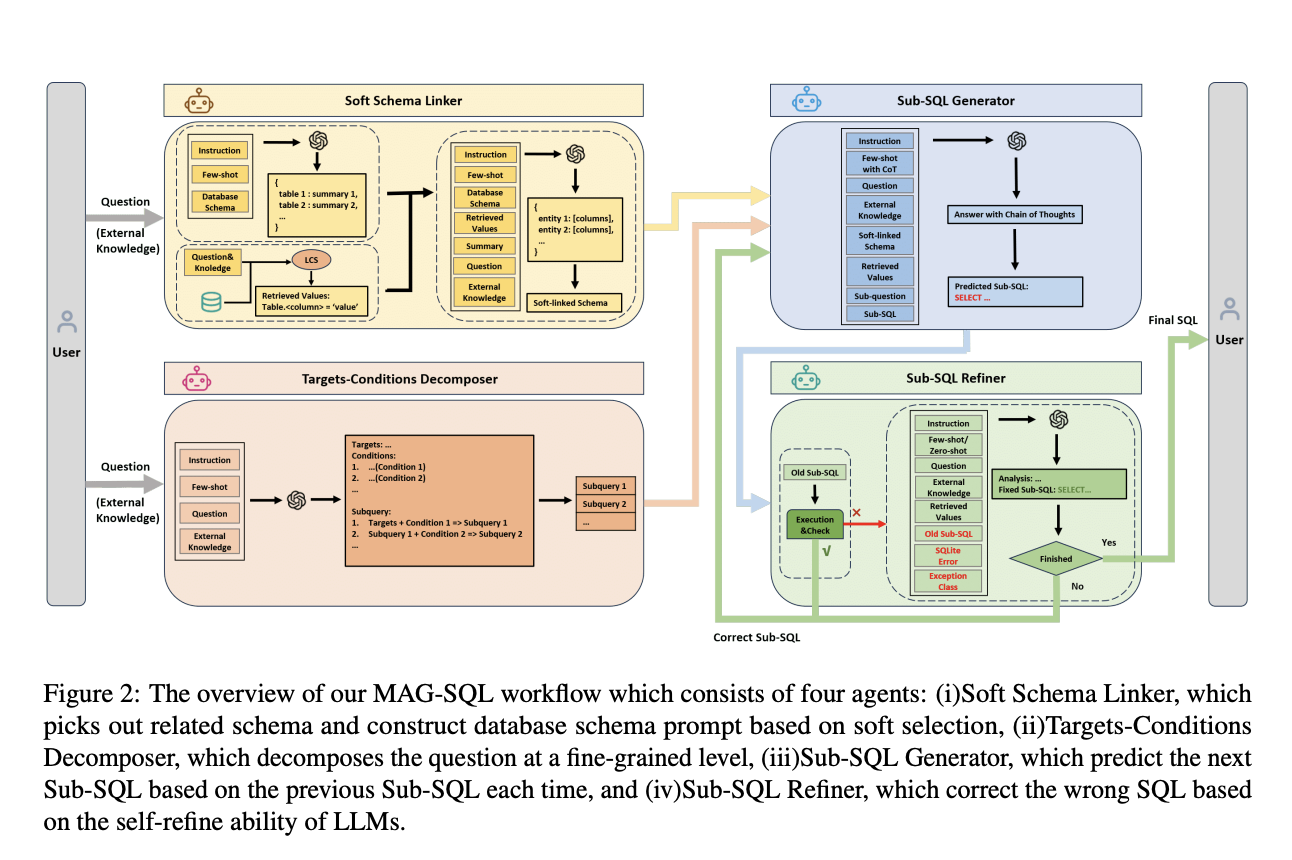
“`html
Text-to-SQL Conversion: Enhancing Data Accessibility and Usability
Text-to-SQL conversion is a crucial aspect of Natural Language Processing (NLP) that enables users to query databases using everyday language rather than technical SQL commands. This process is highly significant as it allows individuals to interact with complex databases seamlessly, regardless of their technical expertise.
The Challenge of Translating Natural Language into SQL
The difficulty in translating natural language into SQL stems from several factors, including the complexity of database schemas and the multifaceted nature of user queries. Many existing methods need help to cope with these challenges, leading to a considerable disparity between the performance of these models and that of humans, particularly on demanding datasets like BIRD.
Introducing MAG-SQL: A Multi-Agent Generative Approach
A research team from South China University of Technology and Tsinghua University introduced MAG-SQL, a novel multi-agent generative approach designed to enhance the Text-to-SQL process. This innovative method combines multiple agents working collaboratively to improve the accuracy of SQL generation. The MAG-SQL framework includes a Soft Schema Linker, Targets-Conditions Decomposer, Sub-SQL Generator, and Sub-SQL Refiner, each crucial in refining the SQL queries generated from natural language inputs.
Performance and Effectiveness of MAG-SQL
MAG-SQL’s performance on the BIRD dataset highlights its effectiveness. When tested using GPT-4, MAG-SQL achieved an execution accuracy of 61.08%, a notable improvement over the 46.35% baseline accuracy of vanilla GPT-4. Moreover, even when using GPT-3.5, MAG-SQL outperformed the MAC-SQL method with an accuracy of 57.62%, demonstrating its robustness and the significant potential of the multi-agent generative approach.
Implementing AI Solutions for Business Growth
Если вы хотите, чтобы ваша компания развивалась с помощью искусственного интеллекта (ИИ) и оставалась в числе лидеров, грамотно используйте MAG-SQL: A Multi-Agent Generative Approach Achieving 61% Accuracy on BIRD Dataset Using GPT-4 for Enhanced Text-to-SQL Query Refinement.
Проанализируйте, как ИИ может изменить вашу работу. Определите, где возможно применение автоматизации: найдите моменты, когда ваши клиенты могут извлечь выгоду из AI.
Определитесь какие ключевые показатели эффективности (KPI): вы хотите улучшить с помощью ИИ.
Подберите подходящее решение, сейчас очень много вариантов ИИ. Внедряйте ИИ решения постепенно: начните с малого проекта, анализируйте результаты и KPI.
На полученных данных и опыте расширяйте автоматизацию.
Если вам нужны советы по внедрению ИИ, пишите нам на https://t.me/itinai. Следите за новостями о ИИ в нашем Телеграм-канале t.me/itinainews или в Twitter @itinairu45358.
Попробуйте AI Sales Bot https://itinai.ru/aisales. Этот AI ассистент в продажах, помогает отвечать на вопросы клиентов, генерировать контент для отдела продаж, снижать нагрузку на первую линию.
Узнайте, как ИИ может изменить ваши процессы с решениями от AI Lab itinai.ru. Будущее уже здесь!
“`