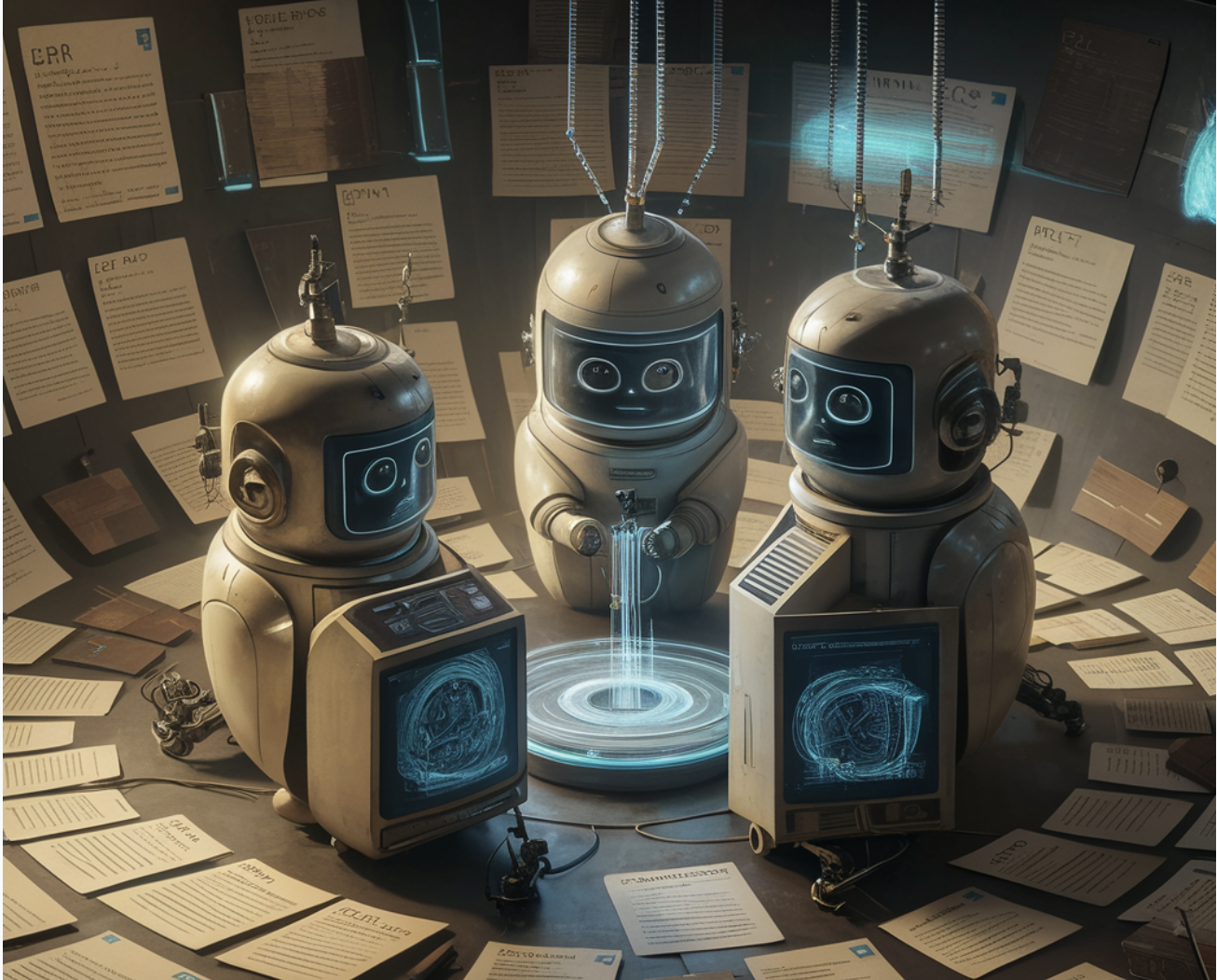
“`html
Retrieval-Augmented Generation (RAG): Providing Relevant Context
RAG combines retrieval mechanisms with generative models to ensure accurate and contextually relevant information from large language models (LLMs). By integrating an external knowledge base, RAG enhances the model’s ability to fetch and integrate pertinent data, minimizing the risk of generating inaccurate information. This approach is beneficial for specialized queries requiring up-to-date or domain-specific knowledge, ensuring grounded and verifiable responses.
Agentic Functions: Ensuring Functional Efficacy
Agentic functions allow LLMs to invoke predefined function calls to perform specific tasks, transforming the model from a passive information provider to an active problem solver. By integrating these function calls, the model’s outputs become informative and actionable, significantly enhancing its practical utility in real-world applications.
Chain of Thought (CoT) Prompting: Facilitating Model Planning
CoT prompting encourages the model to think and plan before generating a response, ensuring accurate and well-reasoned answers. This method is useful for complex problem-solving scenarios, building trust and reliability in the generated responses.
Few-Shot Learning: Leveraging Examples for Improved Performance
Few-shot learning provides the model with several examples to tailor its output to specific contexts or styles, enhancing its adaptability and responsiveness to diverse requirements.
Prompt Engineering: The Art of Effective Communication
Prompt engineering involves crafting prompts to elicit the best possible responses from the model, dramatically improving the relevance and clarity of the model’s outputs.
Prompt Optimization: Iterative Refinement for Best Results
Prompt optimization involves the iterative refinement of prompts to discover the most effective ones, ensuring that the model consistently performs at its peak.
Conclusion
The discussed tools and techniques, such as RAG, agentic functions, CoT prompting, few-shot learning, prompt engineering, and prompt optimization, are indispensable for enhancing the performance of large language models. These methods can ensure that AI outputs are relevant and reliable, delivering clear, actionable, and trustworthy insights in an increasingly complex information landscape.
Sources:
https://arxiv.org/abs/2005.11401
https://arxiv.org/abs/2201.11903
https://arxiv.org/abs/2005.14165
https://arxiv.org/abs/2303.05658
https://arxiv.org/abs/2107.13586
The post Sharpening LLMs: The Sharpest Tools and Essential Techniques for Precision and Clarity appeared first on MarkTechPost.
Контакты:
Пишите нам на https://t.me/itinai
Следите за новостями о ИИ в нашем Телеграм-канале t.me/itinainews или в Twitter @itinairu45358
Попробуйте AI Sales Bot https://itinai.ru/aisales
Узнайте, как ИИ может изменить ваши процессы с решениями от AI Lab itinai.ru будущее уже здесь!
“`