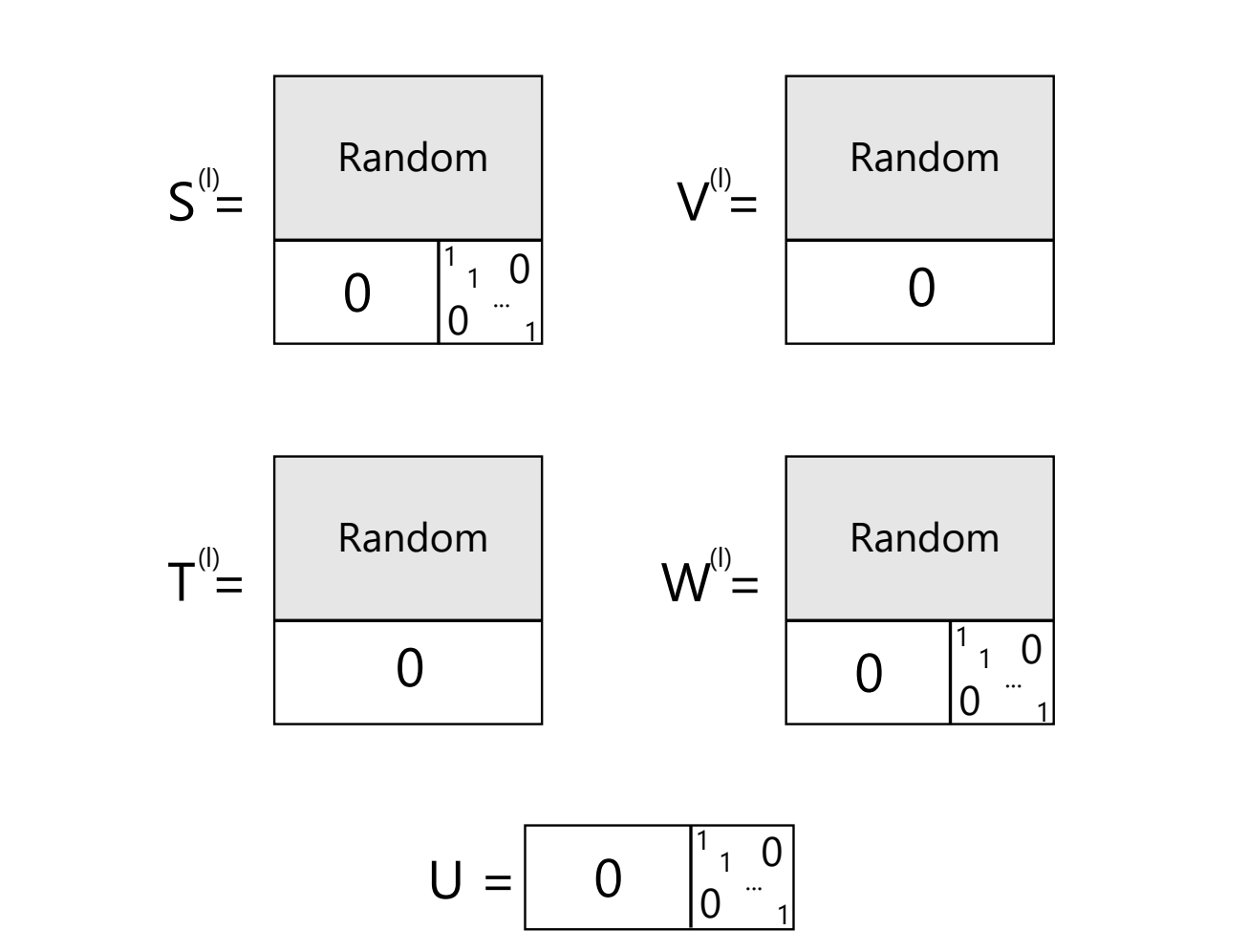
Advanced Machine Learning models: Graph Neural Networks (GNNs)
Graph Neural Networks (GNNs) are powerful models for processing and analyzing graph-structured data. They have been successful in applications such as recommender systems, question-answering, and chemical modeling.
Practical Solutions and Value:
GNNs, specifically Graph Convolutional Networks (GCNs) and Graph Attention Networks (GATs), have demonstrated exceptional effectiveness in transductive node classification. However, the high computational cost of GNNs can be a significant obstacle, especially when dealing with large graphs.
In order to address this challenge, researchers have developed training-free Graph Neural Networks (TFGNNs) which utilize the concept of “labels as features” (LaF) during transductive node classification. This approach allows for more informative node embeddings and enables the model to perform well even in the absence of a traditional training procedure.
Experimental studies have shown that TFGNNs outperform traditional GNNs in a training-free environment, converging faster and requiring fewer iterations to achieve optimal performance. These characteristics make TFGNNs a compelling solution for graph-based applications, particularly in scenarios where rapid deployment and low computational resources are crucial.
Primary Contributions:
The primary contributions of this research include the discussion of “labels as features” (LaF) for transductive learning, formal demonstration of the increased expressive power of GNNs with LaF, and the introduction of TFGNNs as a training-free approach that can function effectively without extensive training.
Join Us:
For more information and to explore the research further, check out the Paper and GitHub. Additionally, stay updated by following us on Twitter, joining our Telegram Channel, and connecting with us on LinkedIn.
Stay Informed:
For further AI insights and discussions, join our ML SubReddit and explore upcoming AI webinars here.
The post Training-Free Graph Neural Networks (TFGNNs) with Labels as Features (Laf) for Superior Transductive Learning appeared first on MarkTechPost.
Применение Training-Free Graph Neural Networks (TFGNNs) с использованием меток в качестве признаков (LaF) для более эффективного трансдуктивного обучения
Если вы хотите, чтобы ваша компания развивалась с помощью искусственного интеллекта (ИИ) и оставалась в числе лидеров, грамотно используйте Training-Free Graph Neural Networks (TFGNNs) с Labels as Features (LaF) для более эффективного трансдуктивного обучения.
Проанализируйте, как ИИ может изменить вашу работу. Определите, где возможно применение автоматизации: найдите моменты, когда ваши клиенты могут извлечь выгоду из AI.
Определитесь какие ключевые показатели эффективности (KPI): вы хотите улучшить с помощью ИИ.
Подберите подходящее решение, сейчас очень много вариантов ИИ. Внедряйте ИИ решения постепенно: начните с малого проекта, анализируйте результаты и KPI.
На полученных данных и опыте расширяйте автоматизацию.
Если вам нужны советы по внедрению ИИ, пишите нам на телеграм-канале. Следите за новостями о ИИ в нашем Телеграм-канале или в Twitter.
Попробуйте AI Sales Bot. Этот AI ассистент в продажах помогает отвечать на вопросы клиентов, генерировать контент для отдела продаж и снижать нагрузку на первую линию.
Узнайте, как ИИ может изменить ваши процессы с решениями от AI Lab itinai.ru. Будущее уже здесь!